Across the world, varying factions of society seem to be angrier and more divided than ever. But as Anna Demming explains, physicists are doing their best to shed light on what has gone wrong

Aeroplane contrails are being deliberately loaded with an extraterrestrial, disease-causing, silicon-based life form: such was the claim back in 2006 by Bill Deagle, a Canadian medical doctor and self-proclaimed “prophetâ€. “This is a silicon-based life form that is intelligent like bees or ants and it fights back,†he warned. Despite no evidence for silicon-based life, Deagle’s claim has continually resurfaced on social media. In March this year, one Facebook post about it received more than 37,000 likes and 33,000 shares amid a flood of endorsing comments.
Before social media came along, we might have thought that making it easier for people to debate would bring us all together and promote consensus. In reality, the opposite seems nearer the mark: people appear to be angrier, and their opinions more polarized than ever. Social media is widely believed to have helped foment the 2011 UK riots, for instance, and more recently the storming of the US Capitol following the 2020 US presidential election. Naturally, there is a keen interest in understanding what is driving these divisions and what – if anything – can be done about them. As it turns out, physics may have some answers.
The idea of applying physics to describe social phenomena extends back at least as far as the 17th-century philosopher Thomas Hobbes, who attempted to describe the “physical phenomena†of society in terms of Galileo’s laws of motion. In recent decades, the development of physical models has grown into a formal field of research known as “sociophysics†(J. Mathematical Sociology 9 1) – encouraged by what appears to be the successful prediction of election results, the demonstration of how polarized views can take hold, and even proposals for how to de-polarize them.
Dangerously alike
For over a decade, political and social scientists have ascribed an increase in division to the effects of social-media bubbles and echo chambers, and the idea that the absence of interactions with people who oppose our views can make our views more extreme. However, when physicists have modelled social behaviour by, for instance, making opposing views repulsive, they fail to replicate this polarizing effect. Indeed, the models even suggest the outcome would be a greater consensus.
In 2020 physicist Michele Starnini at Polytechnic University of Catalunya, Spain and CENTAI, Italy, and colleagues attempted to settle what is driving polarization, by modelling the strength of the opinions held by people as a function of the strength of the opinions they are directly exposed to (Phys. Rev. Lett. 124 048301). Earlier models were usually based on “constructive opinion dynamicsâ€, where unrestricted modes of interaction would eventually lead to a consensus, even on controversial issues. Their new model, however, introduces the dynamics of radicalization as a reinforcing mechanism, so that connections became more likely between people with like views. Starnini’s work shows how extreme opinions can evolve from moderate initial conditions.
The researchers’ model led to three possible states: a consensus, polarized views or radicalized views – the last involving people holding extreme views at one end of the spectrum but not the other (Phys. Rev. X 11 011012). Their analytical solutions reflected what we see empirically – when people’s views are strongly influenced by others, and the issue at stake is controversial, those opinions become more extreme. Add in a strong tendency for people to form connections with others who are like-minded, and the overall network becomes not radicalized, but polarized (figure 1). Indeed, Starnini’s team found that the modelled polarized distribution of opinions agreed with an analysis of real social-media data from users engaged in debates on abortion, Obamacare and gun control on X (formerly known as Twitter), as well as additional user opinion data taken from other social-media sites, such as Facebook and YouTube.
1 Social structure
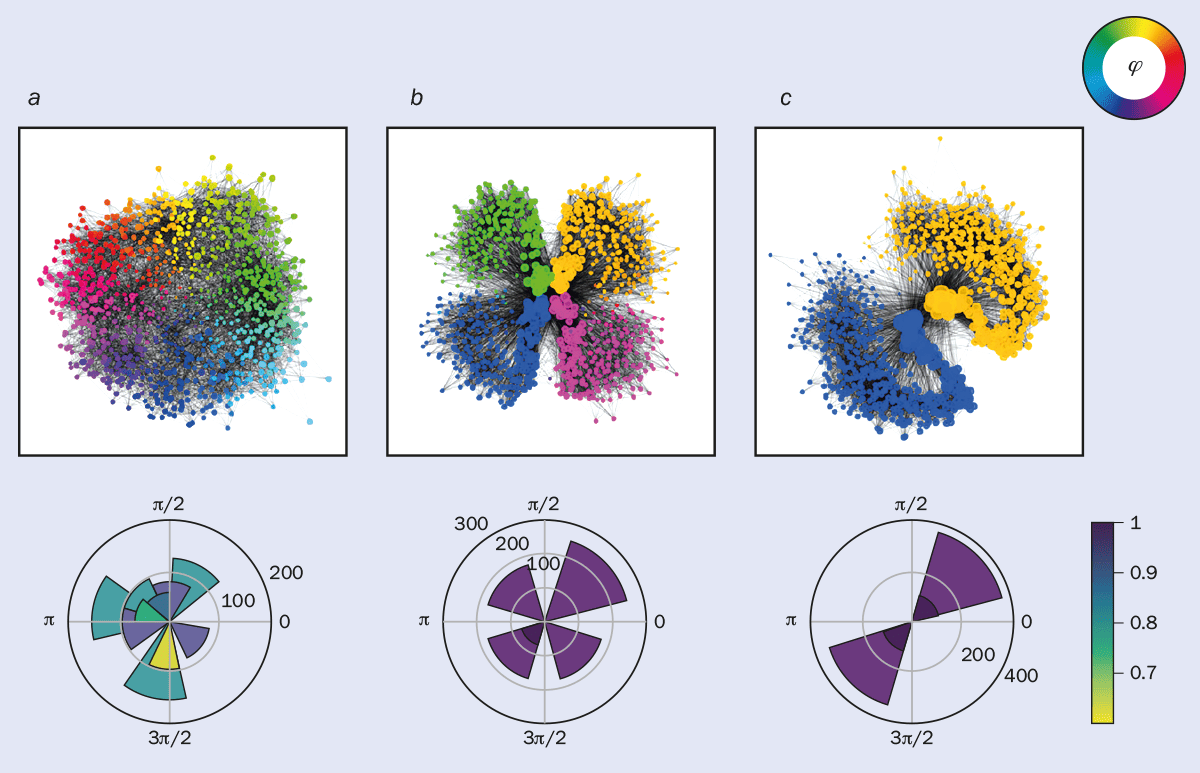
Michele Starnini from the Polytechnic University of Catalunya and colleagues have shown how opinions polarize on social media. Their model indicates opinions on social networks for three different dynamical regimes: (a) approaching consensus, (b) uncorrelated polarized state and (c) ideological state. In the network illustrations (top), each node is coloured according to its opinion angle φ, and its size is proportional to its conviction. Communities that exist within each social network are represented in the polar bar plot below each network, with the radius showing how big it is and the colour and width corresponding to the average cosine similarity between all pairs of agents in the community. The orientation represents the average opinion angle φ of all agents within the community. Communities containing less than 5% of the total number of nodes are not shown.
Their conclusions might seem obvious. But replicating known behaviour in a model is a first step towards less intuitive insights. For instance, Starnini and colleagues’ analysis showed that while the middle ground of opinion is occupied mostly by users with low levels of activity, the extremes are mostly represented by the most active users, as their opinions receive the most energetic reinforcement from like-minded individuals.
Polarized to depolarized – a phase transition
Social-media data have given other insights into increasing polarization. In January 2023 a group including Chaoming Song, a statistical physicist from the University of Miami, along with his student Jiazhen Liu, compared the strengths of user opinions on Facebook posts related to “hard†content (politics) and “soft†content (sport and entertainment). As expected, they found that hard-content opinions are clustered around the extremes, reflecting a polarized state, whereas soft-content opinions are more weighted towards the middle ground (Phys. Rev. Lett. 130 037401, using data collected for Science 348 1130) (figure 2).
Crucially, Song and colleagues showed that these opinion distributions follow a scaling law. There are three stable phases: a converging of opinion on the centre ground; a spreading of opinion evenly across the spectrum with a slight increase, or “partial polarizationâ€, towards the extremes; and full-on polarization. Framing these opinion distributions as phases introduces a potentially valuable concept when looking at the key question of how these opinion distributions might change: the phase transition. Song and colleagues were able to show how phase transitions in online social behaviour – just like those in condensed-matter physics – are characterized by critical points in an order parameter, in this case the most probable or commonly held opinion.
2 Passing phase

Chaoming Song from the University of Miami and colleagues have shown how the evolution of opinion distributions can be described in terms of phase transitions. In this phase diagram, the parameter g is the rate at which opinions align multiplied by the ratio of connections being created versus those destroyed. The parameter J+–J– is the difference between the strength with which like-minded users tend to form connections and the strength with which we tend to break connections with users who hold different views. The red square indicates hard content (HC), such as politics, on Facebook; and the red triangle the soft content (SC) such as sport and entertainment.
Keen to understand how a shift towards depolarization might manifest itself, Starnini and colleagues devised another model that showed how the nature of the phase transition depends on whether an opinion on one topic is correlated, or not, to opinions on other topics (Phys. Rev. Lett. 130 207401). Turning to polls conducted by the American National Election Studies, the researchers identified several statements to which responses appeared to be correlated based on the distribution of the opinions expressed, such as “Religion [provides] guidance in day-to-day living†and “Business owners are allowed to refuse services to same-sex couples if they violate their religious beliefsâ€. But the same poll also provided statements that appeared to elicit uncorrelated responses, such as “Children of unauthorized immigrants born in the US should automatically get citizenship†and “The US should send troops to fight Islamic militantsâ€. The researchers then modelled how such distributions of opinions would evolve in response to the opinions of everyone else in the network, taking into account the “strength of social influence†and how stubbornly those opinions were held.
They found that when opinions are correlated, the transition from a polarized to a depolarized (i.e. consensus) phase is likely to be smooth, like the second-order phase transition that occurs between different magnetic phases in condensed matter. On the other hand, the model showed that when opinions are uncorrelated, the transition is likely to be abrupt, like the first-order phase transition from a liquid to a gas.
In 2015 sociologists Daniel DellaPosta and colleagues at Cornell University in the US provided evidence that political partisanship is increasingly defining the views of individuals across a host of seemingly unconnected areas – from leisure activities and aesthetic taste, to food consumption and personal morality. Crudely, for instance, you can say that liberals like lattes, while conservatives like bird hunting (American Journal of Sociology 120 1473). If our views are becoming more correlated, as DellaPosta and colleagues suggest, we might expect smoother, more second-order phase transitions from polarized to unpolarized states.
The physics of public opinion
But what could initiate depolarization in the first place, and would that be desirable? One could argue that a monoculture of universally held opinions is not great as it’s unlikely to embrace any diversity or deviation from the norm. According to DellaPosta, social and political theorists have long found that arguments favour “cross-cutting lines of conflict and disagreement found in pluralistic societies†(American Sociological Review 85 507). So how then can we reach a more tolerant society? Here, fortunately, a different field of physics has some interesting points to make.
Interventions to unite
Earlier this year, physicist Neil Johnson and colleagues at George Washington University in Washington DC formulated an equation to model the evolution of online “anti-X†communities, where X can be anything, such as a race, a religion or an ethnicity. The equation bore a glaring resemblance to one of the fundamental equations in fluid dynamics – namely the Burgers’ equation, which describes the evolution of a waveform. The connection makes a lot of sense, since the most common characteristic distinguishing these extremist communities from their more benign counterparts is their emergence as “rogue wavesâ€. No matter what the extremist view – be it people who were garnering ISIS support in 2016, or white nationalists today – they seem to “come out of nowhereâ€, says Johnson. This is likely because they operate for a long time below the radar, before suddenly gaining traction in the mainstream.
In their study (Phys. Rev. Lett. 130 237401) Johnson and colleagues modelled individuals or groups of individuals as vectors, so that their different personal experiences could be imprinted in the vector’s multiple dimensions. The probability of individuals joining a group, or of groups fusing to form a larger group, is then a matter of how well their vectors align (figure 3) – something the researchers call “online collective chemistryâ€. Their model suggests that to slow down the sudden emergence of anti-X groups, the trick is to flood a network with more diverse users, so that like-vectors do not find each other so easily. This advice goes against the use of algorithms that block users who appear to be trouble, since these would actually filter out diversity.
3 Community clusters and collective chemistry

Neil Johnson from George Washington University and colleagues have empirically observed (a) fusion and (b) total fission of communities featuring anti-US hate on Russian social-networking service VKontakte, between day t (yellow) and t+1 (blue). Red nodes represent anti-US communities that later got shut down (total fission), while green nodes are those still not yet shut down as of June 2023. The yellow links point to individuals (white dots) removed from the anti-US community on day t+1; while blue links point to individuals added to the anti-US community on day t+1. Spatial layout results from plots (a) and (b) are close-ups of a fuller network that was mapped using ForceAtlas2, meaning that nodes appearing closer together are more interconnected. Plot (b) also shows that in the case of total fission, very few individuals of that community are simultaneously also members of other communities. (c) Empirically observed clustering of anti-government communities across platforms around the US Capitol riot.
Equally, being too proactive about exposing social-media users to opposing views can backfire. In 2018 a study led by sociologist Chris Bail and colleagues at Duke University in the US suggested that this type of effort to puncture the bubbles of echo chambers can increase polarization (PNAS 115 9216). The following year Johnson, Song and colleagues showed that certain algorithm updates by Facebook could have the same counterproductive effect. Although designed to forge new bonds between users and foster new communities, the updates – they said – were likely to lead to the emergence of isolated extremes. Ultimately, this creates a network that more generally evolves in fits and starts, with a vulnerability to fragmenting (Scientific Reports 9 11895).
Yet confronting differently minded individuals is beneficial in the real world. Not everyone we stumble upon day to day is likely to share our views on everything, but while our views may clash on one issue, we can still find common ground on another. Last year, a study by Petter Törnberg, a computational social scientist at the University of Amsterdam in the Netherlands, showed that this varied and stable “patchwork†of differences is not naturally generated in the virtual world by social media, which balloons our potential pool of contacts so that it is far easier to form like-minded connections. With no intrinsic moderation, our opinions are driven to be less mixed, and more partisan (PNAS 119 e2207159119).
One attempt to develop less-divisive social-media platforms is being driven by Luke Thorburn, who is doing a PhD in informatics at King’s College London in the UK, and Aviv Ovadya, a former computer scientist who now studies the societal implications of emerging technology. Together, they are working on a project called “Bridging Systemsâ€, which is finding new ways to rank social-media posts beyond the usual “likes†and reposts. Instead of unthinkingly confronting people with posts from the opposite side of the opinion spectrum, the idea is to recommend posts in which a difference of opinion is sweetened with some explicit point of commonality – what Thorburn refers to as “diverse approvalâ€. For instance, you might not blithely invite a latte-drinking liberal to connect with a grouse-hunting conservative. But you might invite two people on the opposite sides of the political spectrum to engage based on a mutual interest that transcends the usual party lines – be it condensed-matter physics or a particular sport.
Beyond social media
Like in any area of physics, the key test of a good model is not just explaining existing data, but the ability to make correct predictions, and that is what makes physicist Serge Galam’s work so striking. Now based at Sciences Po in Paris, Galam has been involved in sociophysics since its emergence in the 1970s, when it was strongly rejected by physicists (Physics A 336 49). “Atoms never excited me – but humans, yes!†he says.
His models apply a “majority rule†approach to update the opinions of groups of people to gradually adopt the side of the majority, just as daily group-chats over coffee might gradually sway the opinion of someone with no inherent prejudices. After all, it is less taxing to maintain a position on an issue that coincides with those of the people around you. Indeed, Galam suggests that this draws on the principles of energy minimization – as seen elsewhere in physics, from spin alignment in magnets to the folding of proteins in living cells.
Galam considers discrete opinions where the issue is not the strength of an opinion held, but its essential direction one way or the other – a vote in an election, for example. In the evolution of group opinion, he calls the final proportion of opinions for each side the “attractorâ€. For a simple system tending towards a consensus there are two attractors: everybody sharing one opinion, or everybody sharing its opposite. The result is then determined by the initial proportions of opinions on either side, such that a 50:50 mix of initial opinions is the tipping point, where the final outcome could go either way. For polarization to occur, a group with one established consensus must come into contact with another group with the opposite established consensus.

But the path to consensus is not always straightforward – people have doubts where both choices seem equally acceptable. A selection is then made by “chance†with no argument needed to justify it. Here, Galam hypothesized that indeed this “chance†is biased by the strongest prejudice within a group (European Phys. J. B 25 403), which in turn replicates what he calls “democratic minority spreadingâ€, where the strongest prejudice within a group, rather than the opinion with the most numbers, swings things one way or the other. In fact, Galam suggests such prejudices were the determining factor in the 2016 US presidential elections, in which the Republican candidate Donald Trump beat the Democratic candidate Hilary Clinton. In addition to prejudice, Galam’s model includes the existence of other psychological traits, such as contrarianism, and just being plain stubborn, both of which can shift the attractors and the tipping point (Int. J. Modern Phys. B 31 1742015).
Galam notes that where the psychological trait added is stubbornness, the result is a rigid system, which leads to an atmosphere of hate and rejection. Conversely, when more contrarians are added, the proportion of people in favour of each side of an issue will change – the atmosphere will be more tolerant and accepting – even if they started off the same as in the mix of stubborn people. In a 2023 analysis, Galam describes the distinction in terms of entropy with fluid versus frozen polarization (Entropy 25 622), which highlights how the nature of the individuals in a group can matter more than the distribution of voters one way or the other.
Indeed, given enough contrarians there is no tipping point: the outcome always gravitates to a stable 50:50 proportion of opinions held one way or the other, with no hope of consensus. With enough stubborn people the tipping point disappears again – but this time the attractor shifts to have a majority, rather than a 50:50 proportion. “This creates a disturbing, even unethical viewpoint about what is a winning strategy in a campaign,†says Galam. To win an election, a candidate need not aim to persuade the most voters, but only a handful of the most stubborn – and make them stubborn in their favour.
These insights apply whether it is voting on your ballot paper or with your wallet, and could be really powerful for marketing, advertising and similar kinds of manipulation where knowing the targets of your campaign – the key influencers of the net majority – is half the battle. However, Galam emphasizes that it can also be used to prevent a “deadlockâ€, particularly in view of the difficulties of today’s social dynamics, such as fake news.
“This is like any scientific discovery,†he says. “It can be used in a good way or in a bad way.â€