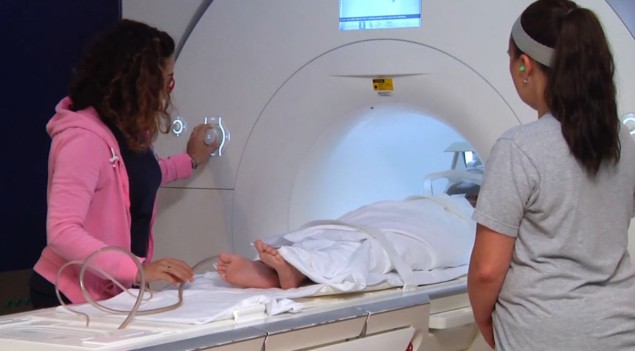
RSNA 2023, the annual meeting of the Radiological Society of North America (RSNA) takes place this week in Chicago, showcasing recent research advances and product developments in all areas of radiology. This year’s event includes numerous papers, posters, courses and education exhibits focused on artificial intelligence (AI) and machine learning applications. Here’s a small selection of the studies being presented.
Ascertaining ADHD traits from brain MRI scans
Attention deficit hyperactivity disorder (ADHD) is a common condition that affects a person’s behaviour. Children with ADHD may have trouble concentrating, controlling impulsive behaviours or regulating activity. Early diagnosis and intervention are key, but ADHD is difficult to diagnose and relies on subjective self-reported surveys.
Now, a research team at the University of California San Francisco (UCSF) has used AI to analyse MRI brain scans of adolescents with and without ADHD, finding significant differences in nine brain white matter tracts in individuals with ADHD.
The researchers employed brain imaging data from 1704 individuals in the Adolescent Brain Cognitive Development (ABCD) Study, including subjects with and without ADHD. From the diffusion-weighted imaging (DWI) data, they extracted fractional anisotropy (FA) measurements, a measure of water diffusion along the fibres of white matter tracts, along 30 major tracts in the brain.
They used FA data of from 1371 individuals as inputs to train a deep learning AI model, and tested the model on 333 patients, including 193 diagnosed with ADHD and 140 without. The AI model found that in patients with ADHD, FA values were significantly elevated in nine white matter tracts.
“These differences in MRI signatures in individuals with ADHD have never been seen before at this level of detail,†says Justin Huynh, from UCSF and the Carle Illinois College of Medicine at Urbana-Champaign. “In general, the abnormalities seen in the nine white matter tracts coincide with the symptoms of ADHD. This method provides a promising step towards finding imaging biomarkers that can be used to diagnose ADHD in a quantitative, objective diagnostic framework.â€
Identifying non-smokers at high risk of developing lung cancer
Lung cancer is the most common cause of cancer death worldwide. In the USA, lung cancer screening using low-dose CT is recommended for current or recent cigarette smokers, but not for “never-smokers†– those who never smoked or smoked very little. Around 10–20% of lung cancers occur in such never-smokers, however, and cancer rates in this group are increasing. And without early detection through screening, never-smokers often present with more advanced lung cancer than those who do smoke.
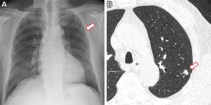
Aiming to improve this situation, a team at the Cardiovascular Imaging Research Center (CIRC) at MGH and Harvard Medical School is testing whether a deep learning model could identify never-smokers at high risk for lung cancer, based on routine chest X-rays. “A major advantage to our approach is that it only requires a single chest-X-ray image, which is one of the most common tests in medicine and widely available in the electronic medical record,†says lead author Anika Walia.
The researchers developed their CXR-Lung-Risk model using 147,497 chest X-rays of 40,643 asymptomatic smokers and never-smokers from the PLCO cancer screening trial. They validated the model in a separate group of never-smokers who had routine chest X-rays. Of 17,407 patients in the study, the model classified 28% as high risk. In six years of follow-up, 2.9% of the total cohort developed lung cancer. Those in the high-risk group far exceeded the 1.3% six-year risk threshold at which screening is recommended.
The team note that after adjusting for age, sex, race and clinical factors, patients in the high-risk group still had a 2.1 times greater risk of developing lung cancer than those assigned to the low-risk group.
Eliminating racial bias in breast cancer risk assessment
Researchers at Massachusetts General Hospital (MGH) have developed a deep learning model that accurately predicts both ductal carcinoma in situ (DCIS) and invasive breast carcinoma using only biomarkers from mammographic images. Importantly, the new model worked equally well for patients of multiple races.
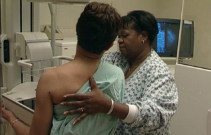
Traditional breast cancer risk assessment models exhibit poor performance across different races, likely due to the population data used to create the model. “Several of the commonly used models were developed on predominantly European Caucasian populations,†explains lead author Leslie Lamb. But according to the American Cancer Society, Black women have the lowest 5-year relative survival rate for breast cancer among all racial and ethnic groups – highlighting the essential need for risk models without racial bias.
In a multisite study, Lamb and colleagues assessed the model’s performance in predicting invasive breast cancer and DCIS, which is early-stage breast cancer, across multiple races. They included 129,340 routine bilateral screening mammograms performed in 71,479 women, with five-year follow-up data. The study group included white (106,839 exams), Black (6154 exams) and Asian (6435 exams) women, as well as those self-reporting as other races (6257 exams) and those of unknown race (3655 exams).
RSNA 2020: AI highlights from an all-virtual annual meeting
The new model consistently outperformed traditional risk models in predicting the risk of developing breast cancer, showing a predictive rate of 0.71 for both DCIS and invasive cancer across all races. The model achieved an area under the ROC curve (AUC) for predicting DCIS of 0.77 in non-white patients and 0.71 in white patients, while for predicting invasive cancer, the AUC was 0.72 in non-white patients and 0.71 in white patients. The team notes that traditional risk models exhibited AUCs of 0.59–0.62 for white women, with much lower performance for those of other races.
“The model is able to translate the full diversity of subtle imaging biomarkers in the mammogram, beyond what the naked eye can see, that can predict a woman’s future risk of both DCIS and invasive breast cancer,†says Lamb. “The deep learning image-only risk model can provide increased access to more accurate, equitable and less costly risk assessment.â€